Travel Technology Analysis: Techniques for Analyzing Travel Data
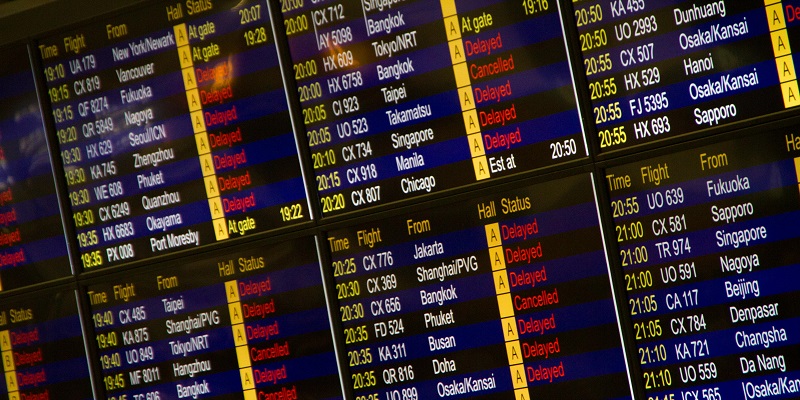
The travel industry has evolved significantly, primarily due to the advancement of technology. With the growing complexity of travel services, companies are increasingly relying on data to understand consumer behavior, optimize services, and drive growth. In this context, travel technology analysis plays a pivotal role. This process involves examining various data sources related to travel, such as booking patterns, traveler preferences, and operational performance. By leveraging advanced analytics techniques, businesses can make data-driven decisions that improve customer satisfaction, enhance operational efficiency, and increase profitability.
The Importance of Data in the Travel Industry
Data is the foundation of decision-making. Travel companies, from airlines to hotel chains, handle vast amounts of data daily. This data comes from bookings, cancellations, customer feedback, social media interactions, and more. Without proper analysis, it becomes impossible to glean any meaningful insights from this sea of information.
Understanding patterns in travel data can help businesses anticipate trends, personalize offerings, and make real-time adjustments to services. For example, airlines can use data analytics to optimize flight schedules, while hotels can adjust pricing based on demand. Effective travel technology analysis allows companies to react to the constantly changing environment of the travel industry, making it an invaluable tool for long-term success.
Key Techniques for Analyzing Travel Data
There are several core techniques that businesses use to analyze travel data. These methods help to uncover patterns, predict future behavior, and make strategic decisions. Below are some of the most commonly used techniques in travel technology analysis:
Predictive Analytics
Predictive analytics is one of the most powerful tools in travel data analysis. This technique uses historical data and statistical algorithms to forecast future outcomes. In the travel industry, predictive analytics can be used for demand forecasting, price optimization, and even determining peak travel times.
By analyzing booking trends, airlines, for example, can predict when flights will likely fill up and adjust prices accordingly. Similarly, hotels can anticipate periods of high or low demand and set dynamic pricing models to maximize revenue. Predictive models help businesses stay ahead of market trends and better allocate resources.
Sentiment Analysis
Customer feedback plays a significant role in shaping travel services. Sentiment analysis is a technique that analyzes customer opinions, reviews, and feedback to understand the general sentiment about a service or product. This process often involves the use of natural language processing (NLP) algorithms that can analyze large volumes of text to determine whether the sentiment expressed is positive, negative, or neutral.
Travel companies use sentiment analysis to improve customer experiences by identifying pain points in their services. For instance, if a particular flight route frequently receives negative feedback, the airline may investigate the cause—whether it’s delays, poor service, or uncomfortable seating—and take corrective action. The insights from sentiment analysis are vital for customer retention and service improvement.
Customer Segmentation
Customer segmentation is another crucial technique used in travel data analysis. This process involves dividing a company’s customer base into distinct groups based on certain characteristics such as travel behavior, preferences, or demographics. By identifying different customer segments, travel companies can tailor their services and marketing efforts to better meet the needs of each group.
For example, a travel company might discover that one group of customers frequently books last-minute flights, while another group prefers to book well in advance. Using this information, the company can develop targeted marketing campaigns for each segment, ultimately driving more bookings and improving customer satisfaction.
Machine Learning and Artificial Intelligence
Machine learning (ML) and artificial intelligence (AI) are becoming increasingly important in travel data analysis. These technologies enable businesses to automate complex data analysis processes and uncover patterns that might not be immediately obvious. In particular, ML algorithms can learn from past data to make predictions and improve decision-making over time.
In the travel industry, AI and machine learning are often used for dynamic pricing, personalized recommendations, and fraud detection. For example, a hotel booking platform might use ML algorithms to recommend the best hotels to a customer based on their previous searches and preferences. AI-powered chatbots are also frequently employed to provide real-time customer service, further enhancing the traveler’s experience.
Real-Time Data Analysis
Travel is a fast-moving industry where circumstances can change rapidly. Real-time data analysis allows businesses to monitor and respond to changes as they happen. Whether it’s adjusting flight prices due to sudden demand changes or rerouting passengers because of delays, real-time analysis provides critical insights that allow companies to make informed decisions on the spot.
This technique is especially useful in sectors like airlines, where operational efficiency is paramount. By constantly monitoring data such as flight schedules, passenger load, and weather conditions, airlines can optimize their operations and minimize disruptions to travelers. Similarly, real-time data helps travel agencies offer last-minute deals, keeping their inventory dynamic and appealing to spontaneous travelers.
Data Sources in Travel Technology
Analyzing travel data involves gathering data from multiple sources. Each source offers a unique perspective on customer behavior and industry trends, making it crucial for businesses to consider a variety of data points. Here are some of the most common data sources used in travel technology analysis:
Booking Data:
Data from online bookings, including details such as travel dates, destinations, and customer preferences, is a key resource for analysis.
Social Media:
Social media platforms are valuable for understanding customer sentiment and gaining insights into travel trends.
Mobile Applications:
Travel companies often gather data from their mobile apps, which provide insights into customer preferences, app usage patterns, and travel behavior.
Customer Feedback:
Surveys, reviews, and customer support interactions offer qualitative data that can be analyzed to improve services.
Operational Data:
Airlines, hotels, and car rental companies generate significant amounts of operational data that can be used to optimize scheduling, pricing, and inventory management.
Challenges in Travel Technology Analysis
While travel data analysis offers immense potential, it also comes with its challenges. One of the primary issues is the sheer volume of data. The travel industry generates a vast amount of data, and without proper tools and technologies, it can be overwhelming to manage and analyze. Additionally, data quality is a significant concern. Inaccurate or incomplete data can lead to misleading insights and poor decision-making.
Another challenge is data integration. Travel companies often use multiple systems for different operations, and integrating data from these disparate sources can be difficult. However, advances in big data technologies and cloud computing are helping businesses overcome these challenges by providing scalable solutions for data storage, management, and analysis.
The Future of Travel Technology Analysis
As the travel industry continues to evolve, so too will the techniques for analyzing travel data. The rise of artificial intelligence, machine learning, and real-time analytics will play an even more significant role in shaping the future of travel technology. Moreover, as customer expectations grow and competition intensifies, companies will increasingly rely on data to offer personalized, seamless travel experiences.
Conclusion
Travel technology analysis is transforming the way companies understand and serve their customers. By leveraging techniques such as predictive analytics, sentiment analysis, and machine learning, businesses can make informed decisions that drive growth and enhance customer satisfaction. As data continues to play a central role in the travel industry, companies that invest in advanced travel analytics will be well-positioned to stay competitive in the years to come.